What is RFM Analysis & Why It Matters for B2C Marketers in 2025?
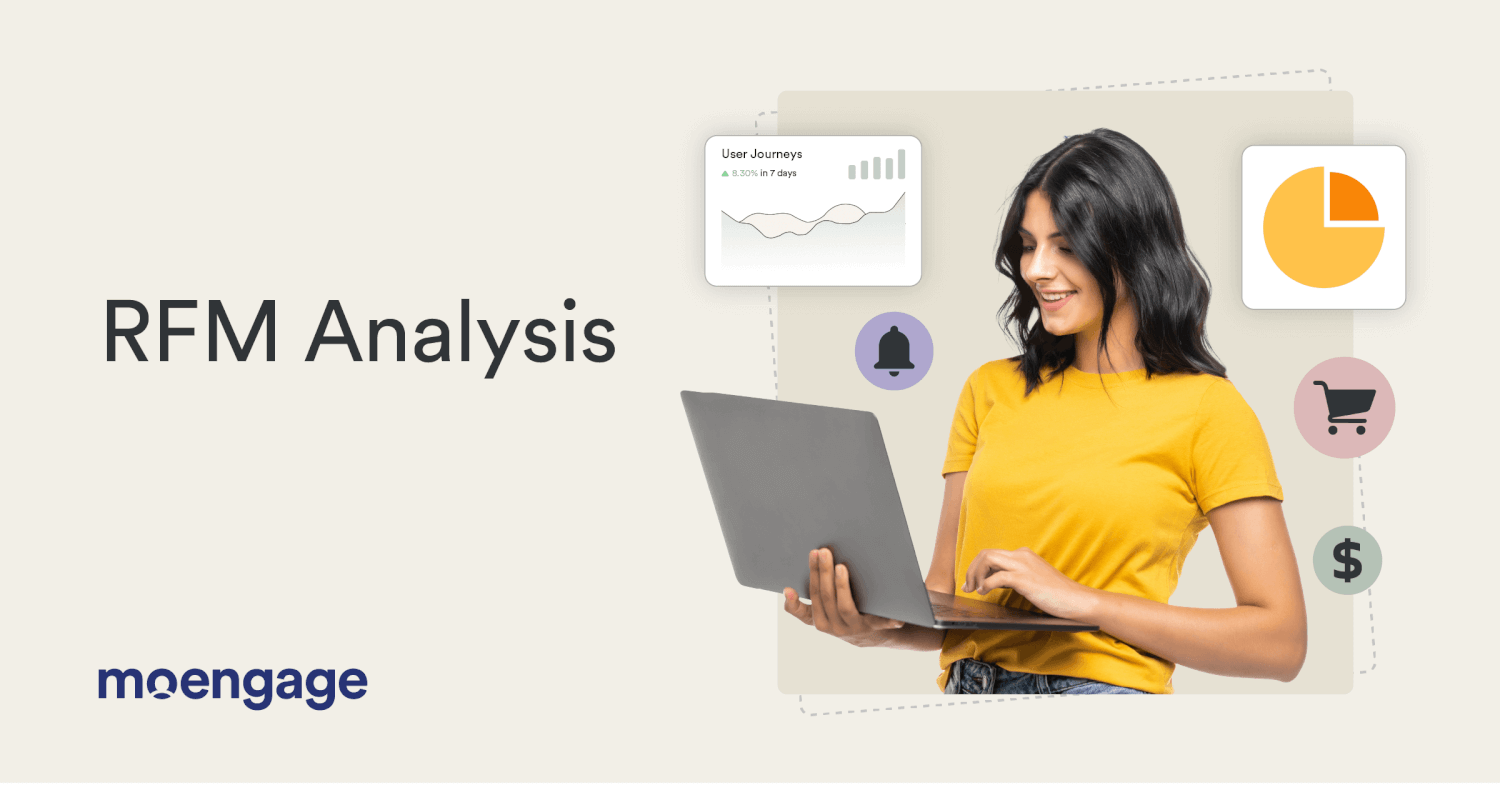
Reading Time: 10 minutes
Have you ever felt like you’re throwing marketing darts in the dark?
We’ve all been there: campaigns that look perfect, have a sleek design, compelling copy, and a discount that seems irresistible. You feel super confident. But the results? Disappointingly flat.
The problem isn’t creativity. It is the approach.
We need to understand that not every customer is the same. Your most loyal customer doesn’t need a massive discount to purchase. But that customer who hasn’t engaged in months requires a completely different strategy.
This means you must create different sub-campaigns targeted at specific audience segments.Â
That’s where RFM comes in— think of it as your marketing GPS.Â
What Is RFM Analysis?
RFM analysis is a customer segmentation technique that evaluates three key metrics: Recency (how recently a customer made a purchase), Frequency (how often they buy), and Monetary value (how much they spend). The technique dates back to 1970.
What Are the Three Components of the RFM Formula?
The “RFM” in RFM Analysis stands for Recency. Frequency. Monetary value. These three variables form the basis for the segmentation.
Using different combinations of these variables, brands can segment customers. For example, they can determine which customers shop the most, which customers are likely to churn, and more to provide them with curated engagement to ensure they’re happy and marketing goals are fulfilled.
The beauty of RFM lies in its simplicity—all the necessary data already exists within your customer database, such as when the customer last visited, last purchased, and more. This is sufficient for the RFM model to function and give results.
Once you implement it, RFM analysis immediately provides you with your most valuable customer segments:
- Ready-to-Buy Customers: Those most likely to make their next purchase soon
- At-Risk Customers: Those showing signs they might leave
- Brand Champions: Your most loyal, high-spending customers
- Long-term Customers: Established patrons who provide consistent value
This data-driven approach helps businesses identify high-value customers, predict purchasing behavior, and develop targeted marketing strategies based on actual customer transaction patterns. RFM segmentation enables marketers to focus on those customer segments with the highest potential for conversions.
With these insights, brands can craft personalized engagement strategies that speak directly to each segment’s specific needs and behaviors—transforming generic marketing into precision-targeted customer experiences.
How to Do RFM Analysis?
RFM analysis assigns scores to customers based on three key variables, helping businesses segment their audience effectively. Let’s see how this works in practice with real examples.
Businesses typically assign scores from 1-5 for each variable (Recency, Frequency, Monetary value). Higher customer activity earns higher scores. These scores can then be combined to create an overall RFM score that guides customer segmentation and targeted marketing efforts.
Let’s understand this better with examples for each variable –
1. What Is Recency in the RFM Model?
Recency measures how recently a customer made a purchase. Customers who’ve engaged with your brand in the last few weeks typically have stronger brand recall and a higher likelihood of purchasing again.
Scoring Example:
- Customer A purchased yesterday – Score: 5
- Customer B purchased 30 days ago – Score: 3
What constitutes “good” recency varies significantly by industry and product lifecycle. A grocery app would view recency differently than a luxury furniture retailer.
2. What Is Frequency in the RFM Model?
Frequency tracks how often customers buy within your timeframe. Higher frequency typically indicates stronger loyalty and engagement.
Scoring Example:
- Customer A made 8 purchases last month – Score: 5
- Customer B made 3 purchases last month – Score: 3
Frequency benchmarks should align with your business model and typical purchase cycles.
3. What Is Monetary Value in the RFM Model?
The Monetary value measures customer spending and often carries the most weight in the analysis. Some customers purchase frequently with low value, while others make infrequent but high-value purchases.
Scoring Example:
- Customer A spent $1,000 in 2 months – Score: 2
- Customer B spent $5,000 in 3 months – Score: 4
The monetary thresholds should reflect your business economics and industry standards.
How to Automate RFM Analysis Using AI Agents?In 2025, doing an RFM analysis is more straightforward and quicker, thanks to AI agents that automatically ingest customer data. MoEngage AI agents can calculate Recency, Frequency, and Monetary values, assigning dynamic scores based on real-time trends. They then create granular customer segments and, leveraging predictive modeling, generate tailored campaign strategies for each segment and more. |
How to Calculate RFM Scores
The first step is to create segment buckets based on the RFM Analysis –
Customers showing similar behavior on R, F, M scores, and RFM aggregate scores are grouped into the same RFM buckets or segments. These segments are identified and named with respect to customer behavior.Â
Here’s the four-step framework to create one such segment bucket –Â
Step 1: Collect and collate relevant Recency, Frequency, and monetary value
Customer | Recency Score (Value) | Frequency Score (Value) | Monetary Score (Value) |
#1 | 1 (10) | 1 (1) | 4 (5500) |
#2 | 2 (8) | 3 (4) | 1 (800) |
#3 | 3 (4) | 4 (7) | 2 (2400) |
#4 | 4 (3) | 5 (11) | 5 (9700) |
#5 | 5 (1) | 2 (2) | 3 (4300) |
Step 2: Set the RFM scales
Score | Recency Scale | Frequency Scale | Monetary Scale |
1 | Last bought a year ago | Bought once a year | Less than 1000 |
2 | Last bought 9 months ago | Bought thrice a year | 1001 – 3000 |
3 | Last bought 6 months ago | Bought 5 times a year | 3001 – 5000 |
4 | Last bought 3 months ago | Bought 7 times a year | 5001 – 7000 |
5 | Last bought 1 month ago | Bought 10 times a year | 7001 – 10000 |
Step 3: Assign RFM scores
Customer | Recency Score (Value) | Frequency Score (Value) | Monetary Score (Value) |
#1 | 1 (10) | 1 (1) | 4 (5500) |
#2 | 2 (8) | 3 (4) | 1 (800) |
#3 | 3 (4) | 4 (7) | 2 (2400) |
#4 | 4 (3) | 5 (11) | 5 (9700) |
#5 | 5 (1) | 2 (2) | 3 (4300) |
Step 4: Label segments
Define clear, descriptive names for each segment that reflect the customer behavior patterns observed in the RFM analysis.
By combining these scores, marketers can identify their most valuable customer segments and tailor engagement strategies accordingly – from win-back campaigns for lapsed high-value customers to loyalty rewards for frequent shoppers.
How to Create RFM Segments Using AI AgentsMoEngage’s AI Agents streamline segmentation, instantly generating different customer segments with a single click. Moreover, these AI agents go beyond segmentation, proactively suggesting tailored campaign strategies for each segment, empowering marketers with actionable insights and accelerated execution. |
Label | Characteristics |
|
These are your dream customers. They’ve purchased recently, shop often, and make substantial purchases. They also create positive WOM (word of mouth) for the brand amongst their circles. Overall, they provide a significant portion of the business’s revenue and must be treated with exceptional attention.
|
|
High-spending customers with the potential to become loyal and contribute significantly to business revenue. |
|
New customers that’ve recently started using the brand and require nurturing to develop long-term brand loyalty. This important segment needs consistent monitoring to prevent customer churn after a few initial purchases. |
|
Customers who’ve recently started using the brand that need nurturing to develop long-term brand loyalty and prevent customer churn after a few initial purchases. This customer segment requires consistent monitoring. |
|
This segment consists of users who may stop using the brand for various reasons. To prevent this, it is essential to examine their behavior and personalize communication further to increase brand trust and loyalty. |
|
This customer segment is vital for any business’s success. These customers have a clear and positive perception of the brand. They are satisfied with the product and service and are unlikely to switch to competitors. It is crucial to make these customers feel valued. |
|
Price is the primary factor influencing the purchasing decisions of these customers, who are always searching for price-led promotions. Their loyalty is not dependent on brand interactions but rather on price fluctuations. This segment can be easily engaged through offers and discounts. |
|
Hibernating customers who have not engaged with the business in a long time are at risk of being lost. The business needs to make a final effort to retain these customers by developing the most relevant and targeted messaging possible. |
|
Existing customers who haven’t made a recent purchase must be encouraged to buy again soon. Otherwise, the brand risks losing the trust and relevance it has built with these customers. |
|
The brand’s existing customers who haven’t purchased recently must be encouraged to buy again soon. If not, the brand risks losing the trust and relevance it has built with these customers. |
How MoEngage’s RFM Segments Help With Customer Segmentation
In MoEngage, each parameter is assigned a value, which ranks customers based on these values. For example, a customer who visited the website one day ago (recency), has made 10 purchases over his lifetime engagement with the website (frequency), and whose last purchase is worth $1,000 (monetary value) would be assigned values of 5, 4, and 4 on the three parameters, respectively. His overall RFM score would be the average of these values at 4.33.
Compared to other segmentation methods, the unique thing about this process is that it is system-driven. Since there’s no manual interference, it significantly reduces errors. Customers with similar scores are categorized into the same segment to enable marketers to target them accurately.
The different segments into which you can bucket customers based on their RFM score are:
- Champions – These are the most recent visitors to the website/app. They are also the most frequent and highest-spend customers.
- Loyal customers – These customers have visited recently, visited often, and made high-value purchases.
- Potential loyalists – These are recent customers who have spent a good amount of money purchasing on the website/app.
Customers falling in the buckets above typically have high RFM scores. But that doesn’t mean you can become complacent with them. You need to monitor their scores and move them to appropriate buckets to ensure that you always engage them effectively.
What customer segments can I create using RFM analysis?
- Recent users – These customers have visited the website/app recently. But they are not frequent visitors, nor has their recent purchase been worth a lot of money.
- Promising – These customers haven’t visited too recently, neither are they among the most frequent or highest value shoppers. They have average scores on all parameters.
- Needs attention – These are customers who have made high-value purchases in the past. However, they haven’t visited the website/app recently.
- Price sensitive – These users have visited recently. They are also frequent but do not spend much money.
- Can’t lose them – These customers have made high-value purchases in the past. However, they do not visit the website/app anymore.
The above categories of customers are candidates for loyalty campaigns. Analyzing their behavior and spending patterns helps to design the right kind of campaigns that they will respond to.
The last two buckets consist of customers who are on the verge of churning out. It is essential to understand them and win them over before they are completely beyond reach –
- About to sleep – These customers have below-average scores on all three parameters.
- Hibernating – These are customers who visited the website/app a long time ago and didn’t spend much.
You can not only bucket customers into the above segments but also view dynamic transitions from one bucket to another.
RFM Analysis Example
Let’s look at a couple of real-life examples of MoEngage RFM Segmentation –Â
1. Bella Vita OrganicÂ
By leveraging MoEngage’s RFM segmentation platform, Bella Vita Organic started re-engaging its dormant customers by disseminating nudges through the WhatsApp channel. This initiative led to a 12X jump in dormant customer conversion!Â
This eventually helped them boost customer loyalty and retention which led to a 57% uplift in the retention rates! Read the complete story here.Â
2. Anteraja
Anteraja, a logistic service based out of Indonesia, used MoEngage’s RFM segmentation model to segment customers based on their in-app activity. Using zero code push templates, they were able to creatively engage customers and improve engagement rates. Read the complete case study here.Â
3. Speedi
Speedi, a leading E-commerce brand based out of Saudi Arabia, utilized the segmentation feature of MoEngage to understand customer behavior and send them messages via push notifications and in-app.
Upon running custom segmentation using RFM, the brand witnessed a higher conversion rate and click-through rates. One of the campaign example that the brand ran using RFM segmentation was for “LOST USERS”. To reactivate these customers, Speedi used their push channel to communicate curated offerings (discount of 10%) and nudge customers to complete action.
Read the complete story here.Â
Benefits of RFMÂ Analysis
By implementing RFM analysis, brands can unlock powerful insights based on actual customer behavior rather than assumptions. This data-driven approach transforms how you engage with different customer segments.
Increased revenue
RFM analysis directly impacts your bottom line by identifying high-value customers and optimization opportunities. By prioritizing engagement with your most profitable segments, you can allocate resources more effectively and drive significant revenue growth.
Streamlined messaging
With clear customer segmentation, you can tailor communications precisely to each group’s behavior patterns. This targeted approach eliminates generic messaging, increasing engagement rates and conversion across all channels.
Enhanced customer lifetime value, customer retention, and customer satisfaction
RFM helps extend customer relationships by revealing exactly when and how to engage different segments. By understanding each customer’s position in their lifecycle, you can implement timely interventions that boost retention and maximize lifetime value.
Steps to Calculate RFM Score
Implementing an effective RFM strategy involves multiple steps. These four steps will help you transition from raw customer data to actionable marketing campaigns.
1. Create a RFM model
Assign scores (1-5) for Recency, Frequency, and Monetary value to each customer in your database. This data can be organized in a spreadsheet or customer engagement platform for analysis.
2. Build customer segments
Divide customers into distinct groups based on their combined RFM scores. These segments reveal behavioral patterns that inform your engagement strategy.
3. Choose segment labels
Name your segments based on their RFM characteristics. Common labels include Champions (high across all variables), Loyalists (high F and M, moderate R), At-Risk (low R, high previous F and M), and Dormant (low across all variables).
4. Develop targeted marketing campaigns for different customer groups
Create specific campaigns for each segment. For Champions, loyalty programs and exclusive offers should be implemented. For at-risk customers, re-engagement campaigns with incentives should be deployed. For Dormant customers, consider win-back campaigns with compelling offers.
Limitations of RFM Analysis
While RFM offers powerful segmentation capabilities, it’s important to understand its constraints. Recognizing these limitations helps brands implement RFM as part of a balanced engagement strategy.
Potential for manual errors
Building RFM models manually introduces a significant risk of calculation errors and misclassification, potentially undermining your entire segmentation strategy. But you can solve that by leveraging MoEngage’s AI Agents.Â
Customer engagement imbalance
Over-focusing on high-value segments may create blind spots, causing you to neglect developing segments with growth potential and creating engagement bias.
Need for complementary approaches
RFM works best as part of a comprehensive segmentation strategy. Combine it with demographic, psychographic, and behavioral data for a more holistic understanding of your customer base.
Is RFM Analysis Still Relevant in the Age of AI?
In 2025, AI agents have revolutionized RFM analysis, transforming it from a manual process to a fully automated, end-to-end solution. These AI agents handle the intricate data ingestion and scoring and autonomously generate and deploy targeted campaigns, significantly reducing the heavy lifting for marketing teams. The simplicity and effectiveness of RFM, now enhanced by AI’s predictive capabilities and real-time data integration, ensure it remains a cornerstone of customer segmentation, adapting dynamically to evolving consumer behaviors and market trends.
Conclusion
RFM analysis offers a proven framework for transforming random marketing efforts into targeted, data-driven strategies. By segmenting customers based on their actual purchasing behaviors, brands can deliver personalized experiences that resonate with each segment’s specific needs. Additionally, they can effectively predict future customer behavior.
While not perfect on its own, when combined with other customer insights, RFM provides an invaluable foundation for building lasting customer relationships and driving sustainable business growth.