E-commerce Product Recommendation Engine – A Beginner’s Guide
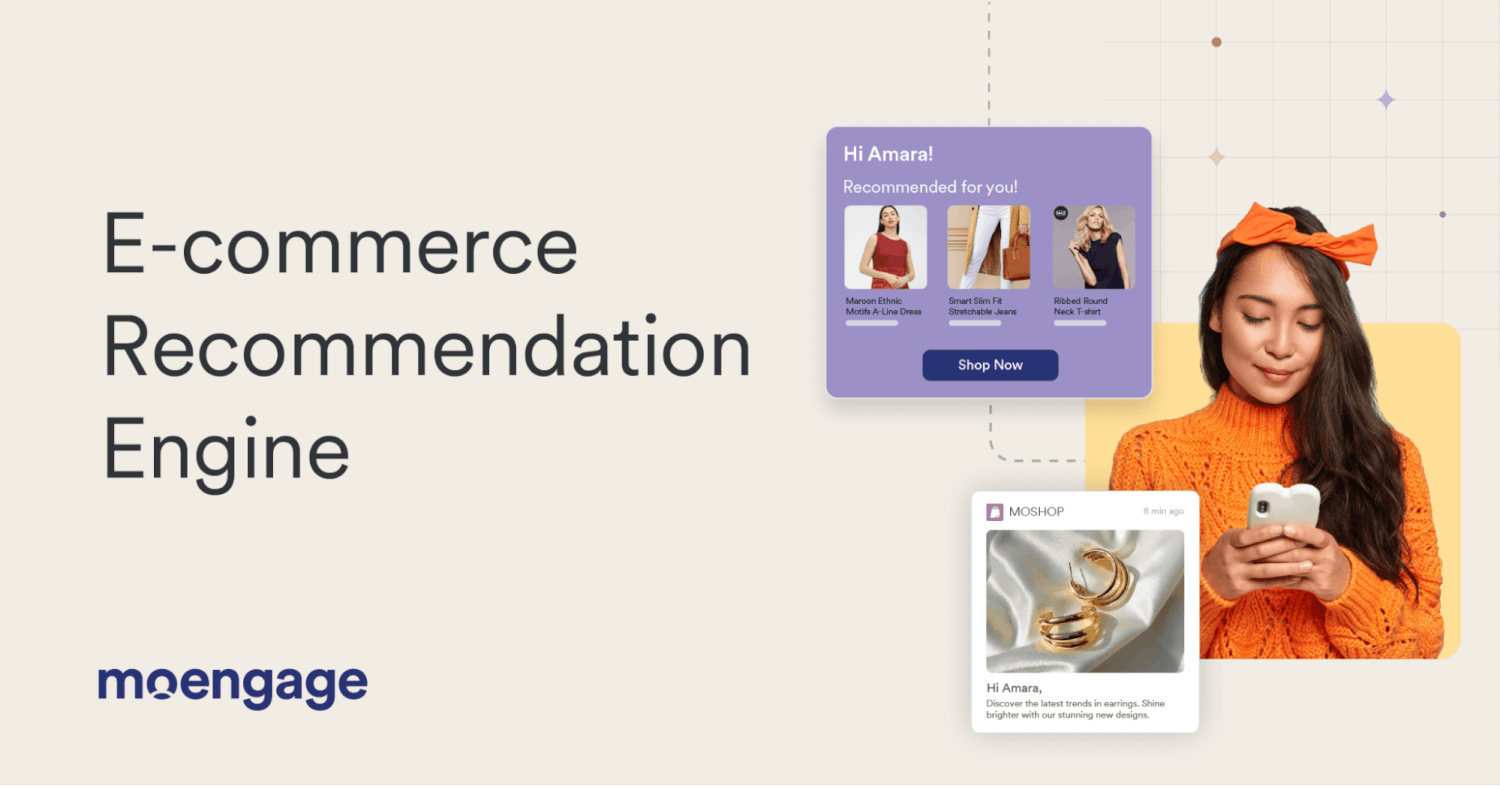
Reading Time: 5 minutes
Product recommendations have become a critical part of the online shopping experience. From helping customers discover new products to influencing their purchasing decisions, E-commerce recommendation engines are crucial in driving sales.
In fact, 35% of Amazon’s E-commerce revenue is attributed to its advanced product recommendation engine.
Over time, these recommendation engines have become more complex in meeting increasing customer demands. However, choosing the perfect recommendation engine can be difficult with so many options available.
But don’t worry—we’re here to help. Our blog will help whether you plan to switch to a better recommendation engine or want to onboard a new one.
In this article, we’ll explore the workings of E-commerce recommendation engines, the various types, their benefits, and more. We’ll also discuss the all-new AI-powered MoEngage Smart Recommendations.
What is a E-commerce Product Recommendation Engine?
E-commerce product recommendations are dynamically generated, real-time personalized product suggestions designed to help shoppers discover the right products they might be interested in buying. These suggestions are usually based on customer preferences, history, previous purchases, trending products, etc.
The recommendations are powered by an E-commerce Product Recommendation Engine.
Types of E-commerce Product Recommendation Engines
Broadly, there are three types of recommendation engines, each using a distinctive filtration method for product suggestions.
Collaborative Filtering
In this type of filtering, customers are added to different segments based on behavior, preferences, and location. The filtering tries to personalize suggestions based on similarities between customers. So, for instance, if a person buys a certain product Y after buying X, all customers who’ve bought X would be recommended Y.
When compared to other recommendation models, its advantage is that it doesn’t require past data or a particular volume of data inputs before it can generate accurate suggestions.
Content-based filtering
This type of filtering suggests products based on previous purchases. For example, if a customer has bought sports shoes, they’ll be suggested other sports clothing like t-shirts, shorts, and more.
The recommendation model focuses on a product’s attributes to suggest similar products. These attributes include color, brand, product category, features, and more.
Hybrid filtering
This method combines collaborative and content-based filtering, which will help customers discover both new products and product categories.
For example, customers who’ve bought running shoes would be recommended sportswear and products from other categories, like smartwatches.
Benefits of E-commerce Product Recommendation Engines
Below are the various benefits of an E-commerce Recommendation Engine –
Boosts Sales
Recommendation engines enable faster product discovery by suggesting the most relevant item to shoppers. This directly helps streamline the shopping experience, enhancing customer satisfaction and increasing sales.
Increases Average Order Value and LTV
Suggesting trending and relevant products during checkout helps in increasing the cart value. This doesn’t boost immediate sales but also increases long-term customer value.
Improves Conversion Rate
Shoppers are more likely to purchase items that match their preferences, and by recommending such products, you’ll increase overall conversions and customer experience.
Reduces Cart Abandonments
Providing relevant product recommendations and nudging customers after cart abandonment help them return back to the app or website, significantly minimizing cart abandonment.
Fosters Loyalty
By providing a personalized experience, brands can establish more trust with their customers, which helps them remain loyal in the long term.
MoEngage Smart Recommendation
Smart Recommendations is our proprietary recommendation engine. We’ve built it to help you create personalized micro-moments for your customers. You can send suitable recommendations to each customer based on their preferences, past interactions, and engagement patterns in real-time, easing product discovery and increasing conversion.
It’s built on seven distinct recommendation models –
Type | Description |
Recommended Items | Offers personalized AI-powered suggestions based on customer’s preferences and past interactions. |
Trending Items Recommendation | Recommends popular products. |
Item Attributes-based Recommendations | Suggests products for specific themes or occasions based on certain defined attributes. |
Customer Action-based Recommendations | Showcases products related to previous customer interactions and wishlist history. |
Similar Items | Recommends products similar to those customers have recently engaged with. |
Frequently Viewed Together | Showcases different relevant categories by suggesting products most commonly viewed together. |
Frequently Bought Together | It helps in maximizing cross-selling by suggesting products that are often bought alongside recent purchases. |
Impact of AI and ML on Recommendations
Artificial intelligence and machine learning capabilities have transformed the landscape of product recommendations. Today’s leading brands harness sophisticated AI-powered recommendation engines that go far beyond the usual “you might also like” suggestions. These systems create highly personalized shopping experiences by accurately understanding and anticipating customer needs.
Here’s a three-step framework that powers the AI Recommendation engines –
1. Data collection
Modern AI systems continuously capture and process numerous data points related to customer interaction. This includes the shopper’s every click, scroll, and pause. The true power of AI lies in its ability to transform seemingly random behavioral data into actionable insights.
The system monitors:
- Product viewing patterns and duration
- Category exploration behaviors
- Purchase history and frequency
- Customer preferences and profile information
- Session-specific interactions
2. Creating customer segments
Based on these insights, AI algorithms create multiple customer segments based on hundreds of dynamic attributes that keep updating according to customer behavior. This dynamic approach ensures that segmentation remains relevant and effective as shopping patterns change.
3. Predictive analytics
The real power of AI recommendation engines lies in their predictive capabilities. By analyzing vast amounts of historical and real-time data, these systems can anticipate customer needs before they arise. This predictive insight enables brands to:
- Forecast seasonal buying patterns
- Anticipate product affinities
- Identify emerging trends within specific customer segments
- Optimize inventory based on predicted demand
The result is a recommendation ecosystem that keeps brands consistently ahead of customer expectations, driving higher engagement and conversion rates while building stronger customer relationships through increasingly relevant suggestions.
Examples of E-commerce Recommendation Engines
1. Amazon
Personalized Search Recommendations
In-app app searches have become more personalized than ever. Brands change the order of search rankings based on your search history, demographic information, or geographic location!
When you go to Amazon and search for sneakers, Amazon shows you results based on your past preferences – your favorite brands and models. The top results make it easier for customers to find what they want!
Dynamic Product Recommendation Grids
If you have ever shopped on an E-commerce app, you must have been recommended many products besides the ones you selected.
These recommendations are based on your behavior patterns (browsing history, past purchases, items added to the cart) or preferences (frequently visited categories, budget range, geographical location, etc.)
Once the system has the data, it analyzes it based on the products that similar customers to you have purchased. It then shows them on a dynamic grid that gets updated based on your real-time browsing and the latest actions on the app.
2. Best Buy
Upsells
If you need to drive upsells its absolutely critical to show the most relevant products. For example, here’s an example from Best Buy where they’re upselling different accessories and insurance plans according to the television.
What’s Next?
E-commerce Recommendation Engines have become a vital part of the online shopping experience. They directly impact your bottom line and customer experience, which is why choosing the right one is incredibly important for your online store’s success.
If you’re interested in learning about our recommendation engine, you can read about MoEngage Smart Recommendations here.